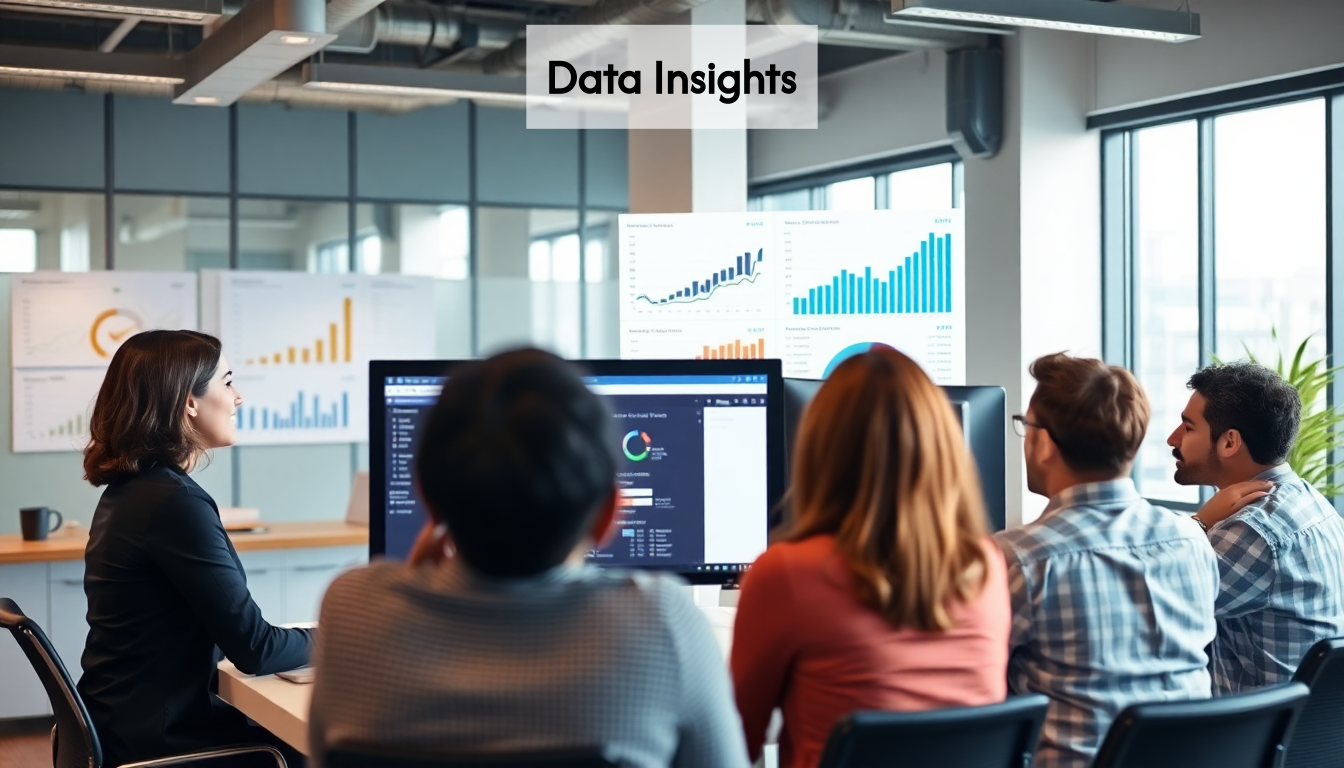
A B Testing Meaning: Uncover Data-Driven Insights for Better Conversions
A B Testing Meaning Unpacked: Definitions and Key Concepts
A/B testing (also known as split testing) is a methodical experimentation process where two or more versions of a variable are shown to different segments of users to determine which performs better against a defined goal. The core purpose of A/B testing is to make data-driven decisions rather than relying on assumptions or intuition.
In its simplest form, A/B testing involves:
- Creating two versions (A and B) of a webpage, email, app interface, or other digital asset
- Randomly distributing these versions to comparable audience segments
- Measuring which version produces better results for your conversion goals
- Implementing the winning version and iterating for continuous improvement
Unlike more complex testing methodologies, A/B testing isolates a single variable, making it easier to identify exactly what influenced user behavior. For example, an e-commerce company might test two different call-to-action button colors to see which generates more sales, while keeping all other page elements identical.
The global A/B testing market is projected to reach $1.08 billion by 2025, highlighting its growing importance in digital marketing strategies. However, only 28% of marketers report satisfaction with their A/B testing performance, suggesting many organizations struggle to implement effective testing frameworks.
Key Takeaways
Takeaway | Explanation |
---|---|
A/B testing isolates variables | By testing only one variable at a time, A/B testing allows marketers to pinpoint what changes influence user behavior directly. |
Importance of statistical significance | Achieving a confidence level of 95% or higher ensures that the results are not due to random chance, making insights more reliable. |
Continuous iteration is key | Successful A/B testing should be an ongoing process, integrating findings into strategies for continuous improvement. |
Real-world impact can be substantial | Minor changes, such as the wording of a button, can lead to significant increases in conversion rates, demonstrating the test's power. |
Adequate sample sizes are critical | Ensuring sufficient traffic volume is necessary to achieve valid and actionable results from A/B tests. |
The Science Behind A B Testing: Methodology & Statistical Insights
A/B testing is fundamentally a scientific experiment applied to digital experiences. Its methodological rigor transforms subjective design choices into objective, data-backed decisions that can dramatically impact conversion rates.
The core methodology follows these essential steps:
- Formulate a clear hypothesis (e.g., "Changing the CTA button from green to red will increase click-through rates")
- Identify your key metrics and success criteria before testing begins
- Randomly assign users to control (A) and variant (B) groups
- Collect data until reaching statistical significance
- Analyze results and implement winning variations
Statistical significance is crucial in A/B testing—it indicates whether observed differences between variants are genuinely due to your changes rather than random chance. Most businesses aim for a confidence level of 95% or higher before declaring a winner, meaning there's less than a 5% probability the observed difference occurred by chance.
Sample size calculation is equally important. Insufficient traffic can lead to inconclusive or misleading results. For example, if your baseline conversion rate is 2% and you're trying to detect a 20% lift, you'll need approximately 25,000 visitors per variation to achieve statistical significance.
While A/B testing seems straightforward, it's essential to understand its statistical foundation. According to Caltech data science researchers, properly designed A/B tests eliminate guesswork by isolating variables and measuring their specific impact on user behavior—transforming intuition-based decisions into evidence-based strategies.
Practical Applications: How A/B Testing Drives Digital Optimization
A/B testing delivers concrete, measurable improvements across virtually every digital touchpoint. Its versatility makes it indispensable for conversion rate optimization across websites, email campaigns, advertisements, and product features.
Here are key applications where A/B testing consistently delivers value:
- Website Optimization: Testing headlines, CTA button colors/text, form fields, pricing displays, product descriptions, and checkout flows to reduce abandonment
- Email Marketing: Comparing subject lines, sender names, content layout, personalization elements, and send times
- Ad Campaigns: Evaluating different ad copy, images, targeting parameters, and landing page combinations
- User Experience: Testing navigation structures, page layouts, interactive elements, and content organization
Real-world impact can be substantial. When Mightybytes conducted A/B tests on button text for a client, changing "Learn More" to "Get Started" increased click-through rates by 30%. This seemingly minor change significantly improved the conversion funnel as documented in their case studies.
Beyond conversion improvements, A/B testing often reveals unexpected insights about customer preferences. For instance, an e-commerce retailer might discover that prominently displaying shipping information on product pages outperforms highlighting discounts—challenging conventional marketing wisdom but providing a data-backed pathway to higher sales.
The true power of A/B testing emerges through consistent application. Rather than a one-off tactic, successful organizations integrate testing into their ongoing optimization strategy, continuously refining digital experiences based on empirical evidence rather than assumptions.
Implementing A/B Tests: Essential Best Practices and Tools
Successful A/B testing requires methodical planning and disciplined execution to generate reliable insights that drive meaningful improvements. Following established best practices significantly increases the likelihood of running tests that deliver actionable results rather than misleading data.
Key Best Practices
- Test one element at a time: A meta-analysis of 2,732 A/B tests revealed that single-variable tests produce more reliable results than multi-variable tests. Isolating changes helps pinpoint exactly what impacts user behavior according to research compiled by Shogun.
- Develop clear hypotheses: Formulate specific, testable predictions about how your changes will affect user behavior and why.
- Calculate adequate sample sizes: Ensure sufficient traffic volume for statistical validity before launching tests.
- Run tests simultaneously: Expose both variants to similar conditions by running them concurrently rather than sequentially.
- Allow sufficient test duration: Most tests require 1-4 weeks to account for day-of-week variation and achieve statistical significance.
- Avoid testing during anomalous periods: Holiday seasons, major promotions, or unusual events can skew results.
Essential Testing Tools
The right A/B testing tools streamline implementation and analysis. Popular options include:
Tool | Best For | Key Features |
---|---|---|
Google Optimize | Beginners | Free tier, seamless GA integration |
Optimizely | Enterprise | Advanced segmentation, multivariate testing |
VWO | Mid-market | User-friendly visual editor, heatmaps |
AB Tasty | E-commerce | AI-powered personalization, advanced analytics |
Choose tools that integrate with your existing tech stack and provide the analytical capabilities necessary for your testing ambitions. Many platforms offer free trials or limited free versions that allow teams to start testing immediately while evaluating long-term needs.
Common Pitfalls and Misconceptions in A/B Testing
Despite its straightforward concept, A/B testing is fraught with potential missteps that can undermine results and lead to incorrect business decisions. Understanding these common pitfalls helps ensure your testing program delivers reliable insights rather than misleading data.
Statistical Misinterpretations
- Confusing statistical and practical significance: Just because a result is statistically significant doesn't mean it's meaningful for your business. A 0.1% improvement might be statistically valid but have negligible impact on revenue.
- Misunderstanding p-values: The p-value is not the probability that your hypothesis is correct; it's the probability of observing your results (or more extreme) if the null hypothesis were true.
- Ending tests prematurely: "Peeking" at results and stopping tests as soon as you see significance dramatically increases false positive rates.
Methodological Errors
- Poor hypothesis formation: Vague hypotheses like "changing the button will improve conversions" lack specificity and reasoning, making it difficult to learn from results.
- Ignoring segmentation: Overall positive results can mask negative impacts on specific user segments, a phenomenon known as Simpson's Paradox. According to experienced growth engineers, failing to segment results by device type, user demographics, or traffic source often hides crucial insights.
- Testing too many variables simultaneously: When multiple elements change at once, you can't determine which change drove the results.
Strategic Oversights
- Treating winners as permanent: Customer preferences evolve, and what works today might not work tomorrow. Successful tests should be periodically re-validated.
- Over-reliance on testing: Not everything requires testing; sometimes user research, expert analysis, or established design principles provide faster, more cost-effective guidance.
- Testing low-impact elements: Focusing tests on minor UI details rather than core user experience or value proposition elements limits potential gains.
By avoiding these pitfalls, organizations can establish more effective testing programs that consistently deliver meaningful improvements to digital experiences and business outcomes.
Future Trends: Innovations Shaping the Future of A/B Testing
While traditional A/B testing remains valuable, technological advances are dramatically expanding its capabilities and applications. The evolution of testing methodologies is creating opportunities for deeper insights and more sophisticated optimization strategies that go beyond simple binary comparisons.
AI-Powered Testing
Artificial intelligence is revolutionizing A/B testing in several key ways:
- Automated hypothesis generation: AI systems analyze user behavior patterns to suggest promising test variables and predict potential outcomes.
- Dynamic allocation: Machine learning algorithms automatically direct more traffic to better-performing variants during tests, maximizing conversion opportunities while experiments run.
- Personalized experiences: Moving beyond one-size-fits-all testing to deliver tailored experiences based on user segments and behaviors.
Advanced Testing Methodologies
Beyond traditional A/B testing, more sophisticated approaches are gaining traction:
- Multivariate testing: Testing multiple variables simultaneously to understand complex interactions between elements.
- Bandit algorithms: These adaptive approaches balance exploration (testing new variants) with exploitation (showing the currently best-performing variant).
- Sequential testing: Allowing for continuous monitoring with rigorous statistical methods that maintain validity despite multiple analysis points.
Cross-Channel Optimization
The future of testing extends beyond isolated webpage elements to unified cross-channel experiences:
- Omnichannel testing: Coordinated testing across websites, mobile apps, email, and other touchpoints to create cohesive user journeys.
- Integration with offline data: Connecting digital test results with in-store behavior or call center interactions for comprehensive optimization.
These innovations are transforming A/B testing from a tactical website optimization tool into a strategic driver of business growth. Organizations that adopt these advanced approaches gain competitive advantages through more sophisticated understanding of customer preferences and behaviors.
Frequently Asked Questions
What is A/B testing?
A/B testing, also known as split testing, is a method of comparing two versions of a variable to determine which one performs better in achieving a specific goal, such as increasing conversions.
How does A/B testing work?
A/B testing works by randomly showing different versions (A and B) of a webpage or digital asset to users, measuring the performance of each version against predetermined metrics to identify the more effective option.
Why is statistical significance important in A/B testing?
Statistical significance ensures that the observed differences between the test variants are not due to random chance. A commonly accepted confidence level is 95%, meaning that the results are highly reliable.
What are common mistakes to avoid in A/B testing?
Common mistakes in A/B testing include ending tests prematurely, testing too many variables at once, and failing to segment user data. These errors can lead to misleading results and ineffective decision-making.
Unlock the Power of A/B Testing with Stellar
Are you tired of relying on guesswork for your marketing decisions? According to the article on A/B testing, statistical significance and isolating variables are critical for uncovering actionable insights that drive conversions. This is where Stellar comes into play. With our no-code visual editor, you can easily set up A/B tests without any technical expertise, allowing you to focus on what truly matters—boosting conversions and enhancing user experiences.
Don’t let complex tools hold you back! Discover how our lightweight 5.4KB script keeps your website running smoothly while delivering real-time analytics. Customize your campaigns instantly with dynamic keyword insertion and dive deep into your user behavior with our advanced goal tracking features. It’s time to transform your insights into results—join Stellar today and experience the quickest, most effective A/B testing solution that small and medium-sized businesses are raving about. Visit https://gostellar.app and start your journey with a free plan for under 25,000 monthly tracked users now!
Published: 3/19/2025